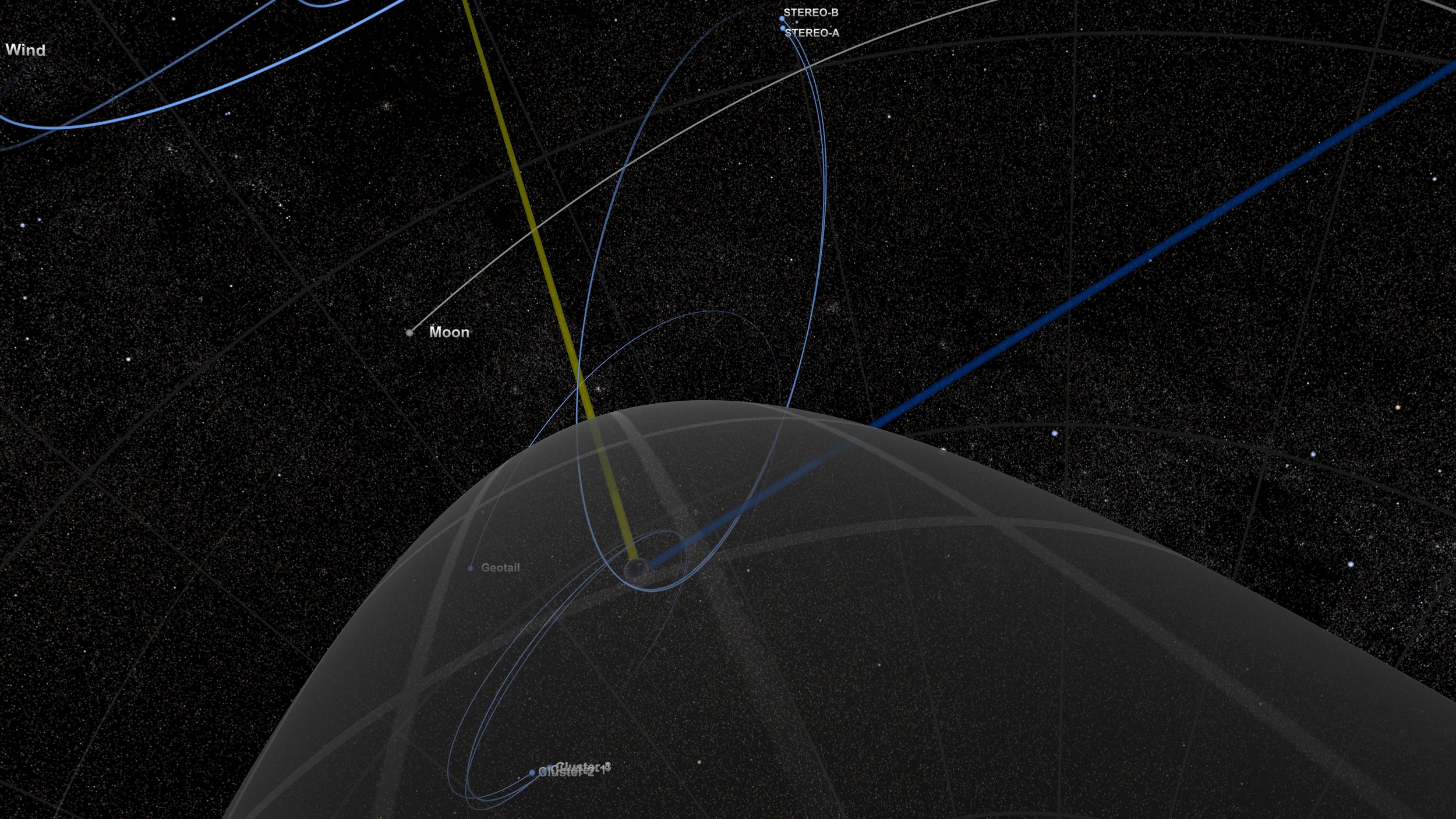
Machine Learning
Predictive models of geomagnetic storms can be broadly divided into two types: physics-based models and statistics-based (or data-driven) models. The first type of models make predictions based on the known physical laws of nature by solving mathematical equations. For instance, magneto-hydro-dynamics (MHD) models are first principles models that simulate plasmas according to the fundamental laws defined by the Navier-Stokes equations of fluid dynamics and Maxwell’s equations of electromagnetism. Although these are based on first-principles and may allow researchers to derive physical insight about a system, they are computationally expensive. Space weather predictions typically require a super computer with thousands of processors to obtain an approximate solution of a geomagnetic storm.
The second type of models, designed to produce faster and more efficient simulations, are statistical or data-driven models that digest large quantities of past observations, make inferences from those data, and apply them to predict the timelines of future events. While they can provide fast predictions that can be used for space and power grid operations, they lack in physical insight and cannot predict events outside the data that was fed to them.
These models are mainly based on linear regression algorithms along with polynomial and wavelet functions.
The explosion in big-data usage seen across many industries in recent years has brought forth huge advancements in machine learning techniques and processing platforms. While these are used extensively to predict the behavior of humans and economic entities, the use of machine learning in scientific inquiry is still an emerging field. The promise of machine learning is that although these too are statistical models that cannot operate outside the data used for their training, is that they may reveal patterns and interconnections within the multi-dimensional data sets prevalent in space physics, and perhaps would also allow for more accurate predictions for operational stakeholders.
Prof. Ilie and her group are developing a decision tree-based machine learning model for the near-Earth space environments. The training data set is provided by the Cluster II mission from ESA. The model could also train on other data sets.